Okay, so yesterday I was messing around with some tennis predictions, specifically the Fritz vs. De Minaur match. Thought I’d document how I went about it, since it was a bit of a rollercoaster.
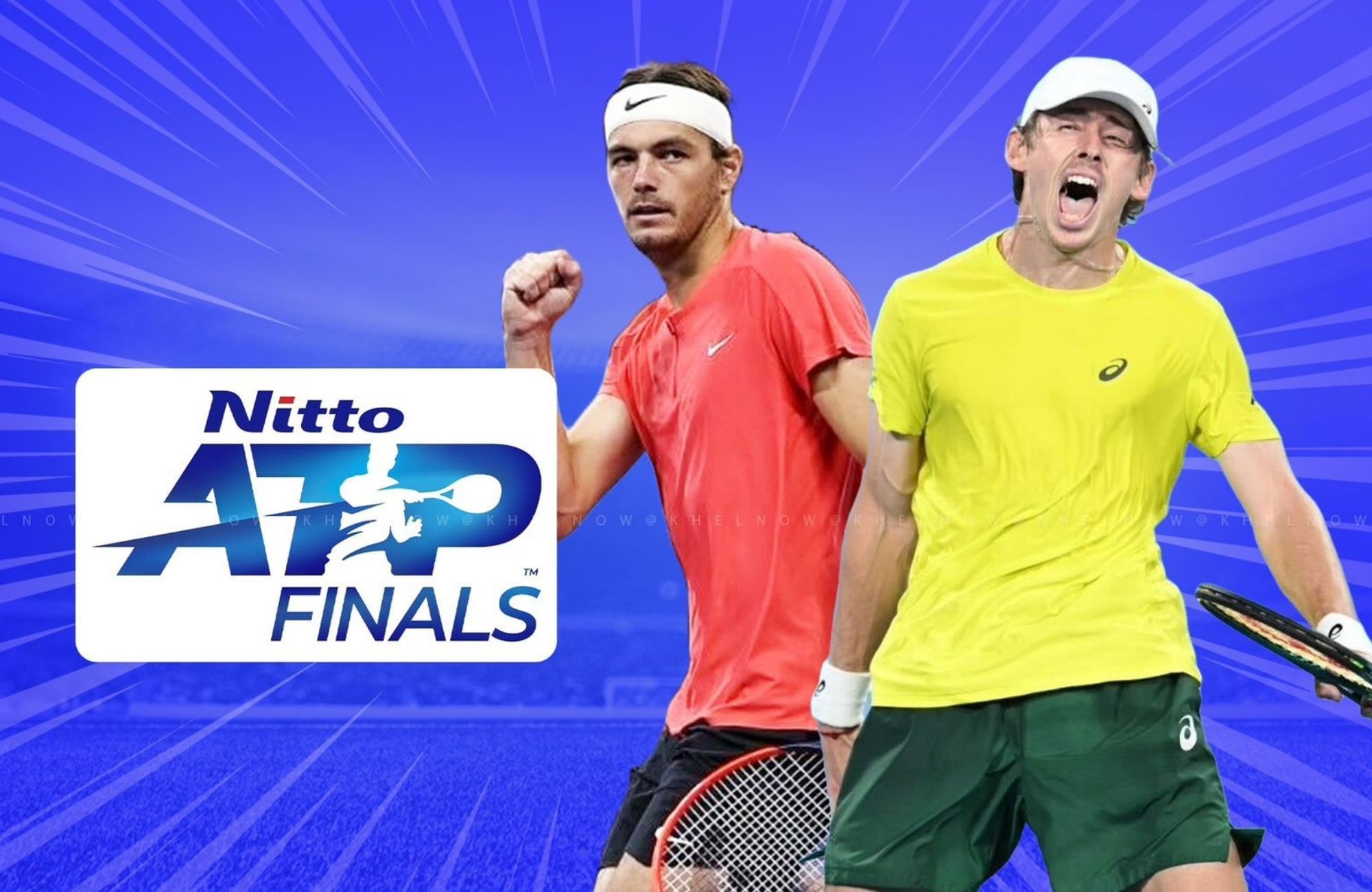
First off, I started by gathering some data. I scoured the internet for recent match stats, head-to-head records, that kind of stuff. Found a few decent sites that tracked that stuff pretty well. I wasn’t aiming for crazy in-depth analysis, just a quick overview of their recent form.
Then, I took a look at their playing styles. Fritz is a big server, likes to dictate play. De Minaur is a grinder, super quick, makes you work for every point. This is where I started forming a gut feeling. I figured if Fritz’s serve was on, he’d be tough to break down. But if De Minaur could get him into rallies, he’d have a good chance.
Now, here’s where things got a little more… involved. I thought, “Hey, let’s try to quantify this somehow.” So I pulled out some old spreadsheets and started tinkering. I tried assigning weights to different factors – serve percentage, return points won, unforced errors, etc. It was pretty rough, just eyeballing it really.
- Gathered match data
- Compared playing styles
- Created a (very) basic prediction spreadsheet
Honestly, the spreadsheet was a mess. But it did give me something to play around with. I tweaked the weights, ran some “simulations” (basically just recalculating the spreadsheet a bunch of times with slightly different inputs), and saw how the projected scores changed.
After all that fiddling, my “model” was leaning slightly towards Fritz, but it was close. Which matched my gut feeling. So I figured, okay, maybe there’s something to this after all.
Of course, the match happened, and… well, it didn’t go exactly as planned. Fritz did win, but it was a real battle. De Minaur made him work for every single point, just like I expected. The scores were tighter than my “model” predicted.
What did I learn? That predicting tennis matches is hard! And that my spreadsheet skills need some serious work. But also, that even a basic, flawed analysis can sometimes give you a decent starting point. It was a fun little project, and I’m already thinking about how to improve my prediction “engine” next time. Maybe I’ll try to incorporate some more advanced stats, or even dabble in some machine learning stuff. We’ll see!