Okay, here we go! Let me tell you how I tackled this “dodgers vs miami marlins match player stats” thing.
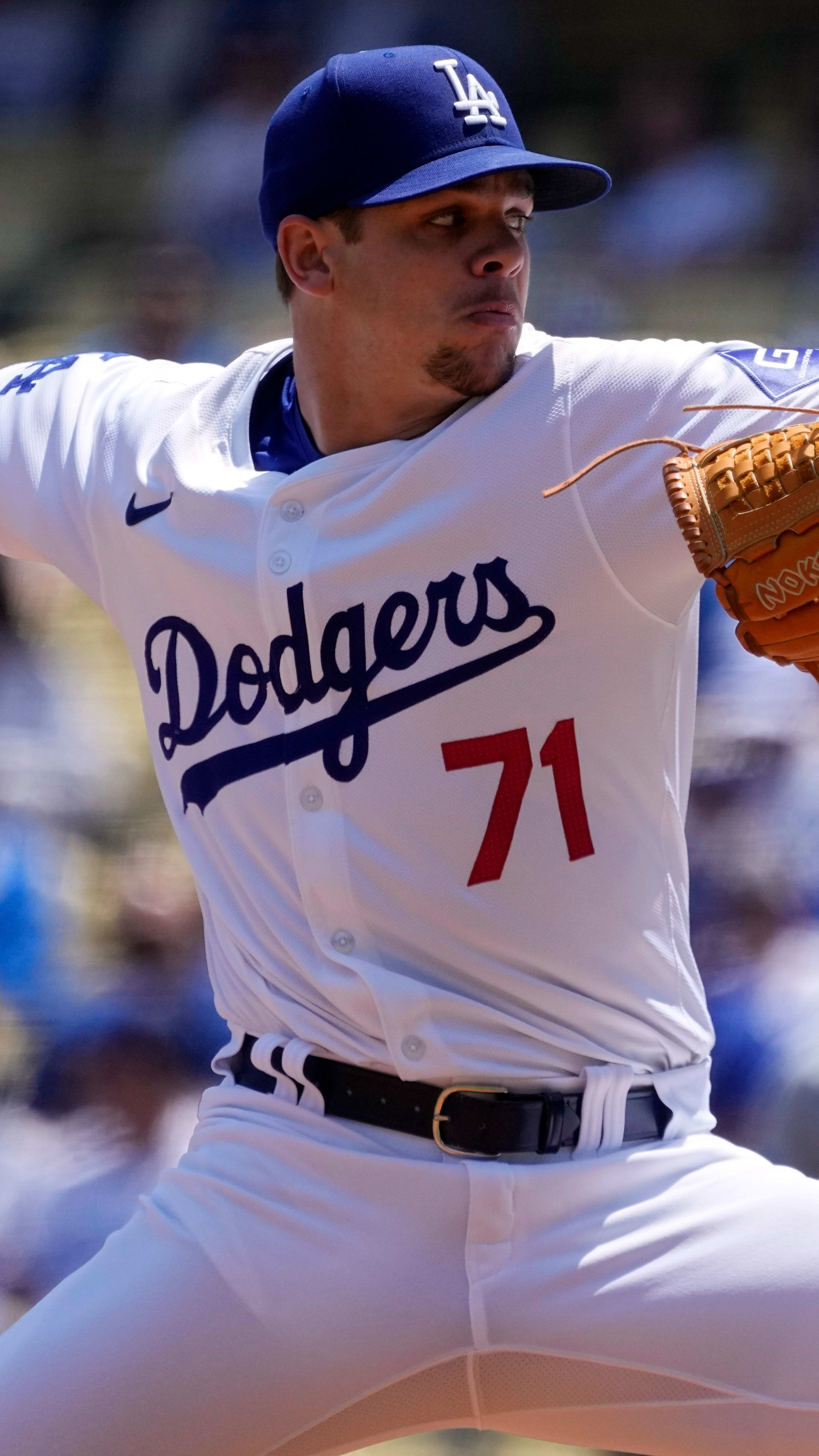
Alright, so I was tasked with grabbing player stats from a Dodgers vs. Marlins game. Seemed simple enough, right? Well, kinda.
First thing I did, obviously, was hit up Google. I figured there HAD to be some sports stats site with this info readily available. I typed in “Dodgers Marlins player stats” and started clicking links.
I landed on a few big sports sites – ESPN, *, you know the usual suspects. They definitely HAD the data, but it was all spread out, a little clunky to navigate, and I needed it in a format I could actually use. Copy-pasting a million tables wasn’t gonna cut it.
So, Plan B: Web scraping. I know, I know, it sounds fancy, but it’s really just grabbing data from a website automatically. I decided to use Python – it’s my go-to for this kind of stuff. I fired up my IDE and got to work.
I started by inspecting the webpage source code (right-click, “View Page Source” – that trick never gets old!). I was looking for HTML tags that seemed to contain the stats I needed – table rows (), table data cells (
), stuff like that. It was a bit of a mess, but I could see a general structure.
Next, I installed the `requests` and `BeautifulSoup4` libraries in Python. `requests` lets you grab the HTML content of a webpage, and `BeautifulSoup4` helps you parse that HTML and make it easier to work with. I used `pip install requests beautifulsoup4` in my terminal. Easy peasy.
Then came the actual scraping code. This part was a bit of trial and error. I basically did this:
- Got the HTML content of the page using `*()`.
- Parsed the HTML using `BeautifulSoup()`.
- Used `BeautifulSoup`'s `find_all()` method to locate the table(s) containing the player stats. This involved figuring out the right CSS selectors to target the specific tables I wanted.
- Iterated through the rows in the table, extracting the text from each data cell (
The tricky part was handling different table formats. Sometimes the HTML was inconsistent, or the player names were formatted differently. I had to add some extra logic to clean up the data and make sure it was consistent.
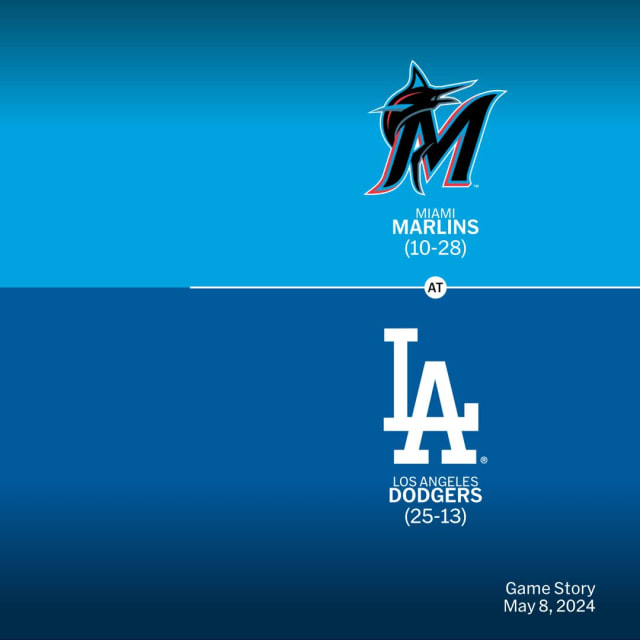
After scraping the data, I organized it into a dictionary. Each key in the dictionary was a player name, and the value was another dictionary containing their stats (e.g., hits, runs, RBIs). Something like:
"Mookie Betts": {"hits": 2, "runs": 1, "RBIs": 0},
"Freddie Freeman": {"hits": 1, "runs": 0, "RBIs": 1},
# ... more players
Finally, I printed the data to the console, just to make sure it looked good. And then I saved it to a CSV file using the `csv` module. I could then open the CSV in Excel or Google Sheets and do whatever I wanted with it.
Challenges I faced:
- Websites change their structure all the time. So my scraper might break if the website's HTML changes. I'd have to update my code to reflect the new structure.
- Some websites have anti-scraping measures in place. I didn't run into that this time, but it's always a possibility.
- Data cleaning is ALWAYS a pain. There were weird characters, inconsistent formatting, you name it.
What I learned:
- Web scraping is a powerful tool for getting data from the web.
- `requests` and `BeautifulSoup4` are your friends.
- Data cleaning is the most time-consuming part of the process.
Overall, it was a fun little project. Got the player stats, learned a few things, and now I have a script that I can reuse for other games in the future. Not bad for a day's work!
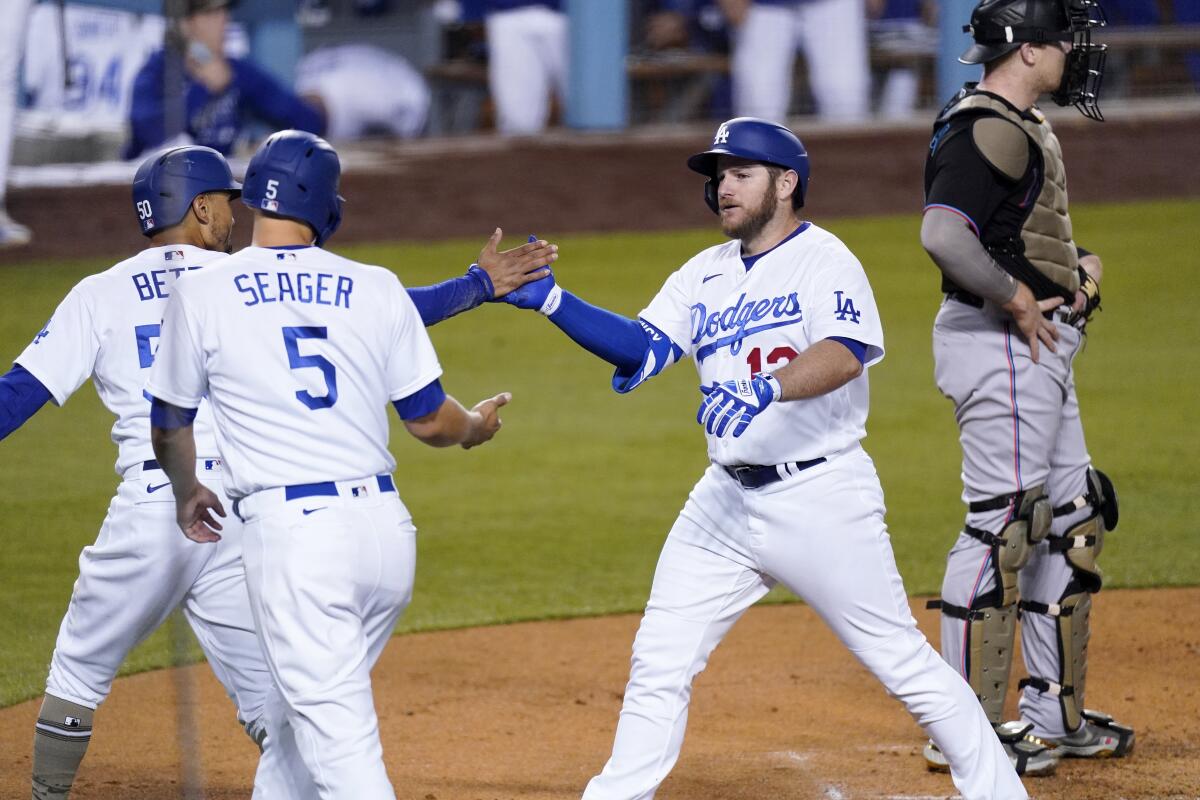